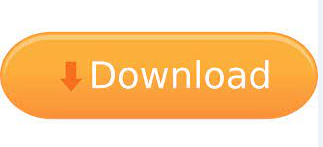

Since then, sports teams in many leagues have established a data analytics department, hiring some of the brightest minds in the field to improve their operations. Michael Lewis’ 2003 novel Moneyball, chronicling Oakland’s season and its use of analytics, launched sabermetrics into the public consciousness and showed that analytics-based strategies can work in the sports world. The turning point was in 2002, when the Oakland Athletics, under the direction of general manager Billy Beane, embraced data analytics and surprised many with a successful season despite a roster of castoffs. These professionals were scorned by the establishment at first, but their methods slowly took hold. Throughout the latter part of the 1900s, SABR researchers refined the statistics already used in baseball and developed their own algorithms to calculate more complex ones.

Founded in 1971, the Society for American Baseball Research (SABR) gave birth to the movement now known as “sabermetrics,” a term coined by Bill James, the movement’s most prominent voice. Continue reading to learn more about analytics in this field, including how data analytics helps in sports, its impact on different aspects of the sports world, and how you can help a sports franchise use analytics to get ahead of the competition.Ī few decades before the turn of the 21st century, analytics and baseball started to mesh. Business executives and operations professionals across sports are embracing the analytics revolution to streamline their businesses and increase profits –– and often wins, too. Now, the conversations are about on-base percentage plus slugging (OPS), wins above replacement (WAR), win probability added (WPA), fielding independent pitching (FIP), and many other statistics that better assess a player’s value.īaseball is not the only sport using big data analytics in the 21st century. Once front offices brought in big data, the sport changed completely. That was it, from the early 1900s all the way to the early 2000s. They judged the best hitters by how many home runs they slugged and how many runs they drove in, and they determined the best pitchers by how few runs they allowed. For most of Major League Baseball’s existence, there were only a few statistics that writers, managers, and owners cared about: batting average, home runs, and runs batted in (RBI) for players, and wins and earned run average (ERA, or the approximate number of runs allowed every nine innings) for pitchers.
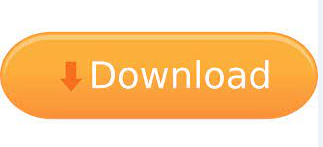